Suhail Sherif Chosen as Recipient of ACM India 2021-2022 Doctoral Dissertation Award
January 14, 2022
Suhail Sherif is the recipient of the ACM India Council's 2021-2022 Doctoral Dissertation Award for his dissertation titled “Communication Complexity and Quantum Optimization Lower Bounds via Query Complexity” that makes breakthrough progress on understanding basic complexity measures.
Suhail completed his doctoral dissertation under the supervision of Arkadev Chattopadhyay at Tata Institute of Fundamental Research, Mumbai.
Suhail’s dissertation makes two distinct and significant contributions. The first contribution sheds new light towards understanding the effectiveness of rank based measures in estimating communication complexity of Boolean functions. The second contribution deals with understanding the relative advantage of quantum algorithms over classical randomized ones for solving certain optimization problems. In both parts, variations of the model of query complexity play an important role.
The principal result in Suhail’s dissertation is a surprising refutation of a well-known conjecture in the area of communication complexity. The Log-Rank Conjecture (LRC) of Lovasz and Saks (1988) is among the most famous open problems in this area. Simply put, the LRC asserts that the amount of communication needed between two deterministic distributed parties to collaboratively solve a (total) task is characterized by a classic linear algebraic quantity: that of the (log of the) rank of an associated matrix. In 2007, a natural analogue of the LRC for randomized computation, called Log-Approximate-Rank Conjecture (LARC) was formalized by Lee and Shraibman. For over a decade, researchers have tried tackling the LARC, proving special cases of it, etc. Suhail’s dissertation strongly refutes the LARC. His result has already led to several important follow-up work by others, including disproving the quantum analogue of the LARC, and improvements and optimizations of Valiant’s classical result showing the power of negations in the context of algebraic computations.
The other major result in Suhail’s dissertation concerns convex optimization and quantum algorithms. Specifically, Suhail showed that the classical gradient descent algorithm, used widely in deep neural networks, cannot be significantly sped up on a quantum computer. This area is pivotal for the understanding of deep neural networks, and gradient descent is the algorithm of choice for optimization. Suhail’s result showing that this cannot be run much faster on a quantum computer therefore has significant ramifications.
Suprovat Ghoshal is recipient of the Honorable Mention of the ACM India Council's 2021-2022 Doctoral Dissertation Award for his dissertation titled “New Algorithmic and Hardness Results in Learning, Error Correcting Codes and Constraint Satisfaction Problems” that resolves several questions of interest at the intersection of learning theory, error corrections and combinatorial optimization, and asks new ones which are likely to lead to a better understanding of the complexity of problems in this landscape.
Suprovat completed his doctoral dissertation under the supervision of Arnab Bhattacharyya and Siddharth Barman at Indian Institute of Science, Bengaluru.
The contributions of Suprovat’s dissertation are multifaceted: it settles the complexity of several fundamental problems, and also initiates a systematic study of fundamental but relatively under-explored problems in constraint satisfaction. Specifically, Suprovat establishes tight in-approximability bounds for (improperly) learning concept classes using threshold functions, which is a hypothesis class that generalizes Halfspaces and arises naturally in machine learning theory and practice. While they are widely studied, a well rounded understanding of learnability using thresholds has stayed elusive due to their expressive power. Suprovat’s dissertation makes important progress towards closing the gap by providing tight results for learning natural concept classes such as Halfspaces and Disjunctive Normal Forms (DNFs) that simultaneously unify and strengthen previous state-of-the-art results in this context. The techniques used in his dissertation bypass existing bottlenecks from previous works and employ new analytic and probabilistic tools for polynomials which might be of independent interest. Another key contribution of the thesis is that it establishes the parameter dependent lower bounds for finding sparse solution to linear equations over finite fields, and in particular the Even Set problem, which has been a long standing open question in the world of Fixed Parameter Tractability. The techniques used here played a substantial role in a subsequent work that settled the fixed parameterized intractability of Even Set under randomized reductions.
Suprovat’s dissertation also provides new algorithmic and hardness results for vertex deletion Constraint Satisfaction Problems (CSPs). These are a class of CSPs that express several natural problems in combinatorial optimization such as Odd Cycle Transversal and Strong Unique Games, but are relatively less well understood in comparison to their edge deletion counterparts (namely Max Cut and Unique Games) due to their vertex deletion nature. Suprovat introduces a suite of novel techniques which allow for a systematic treatment of these problems – leading to new approximation and hardness bounds.
Finally, Suprovat’s dissertation also explores the role of property testing in this landscape, introducing new efficient algorithms for property testing variants of (worst case intractable) problems in machine learning such as Compressed Sensing and Dictionary Learning. These results imply yet another way of bypassing the intractability barrier in applications where the search/optimization problem is hard.
Vrunda Dave is co-recipient of the Honorable Mention of the ACM India Council's 2021-2022 Doctoral Dissertation Award for her dissertation titled “On Some Fundamental Problems and Applications of Word Transducers” that makes fundamental contributions to and advances the theory of transducers.
Vrunda completed her doctoral dissertation under the supervision of Shankara Narayanan Krishna at Indian Institute of Technology Bombay, Mumbai.
An important result Vrunda proved in her thesis relates to the synthesis of transducer expressions from two way transducers, and their equivalence. The significance of this result is two fold : one, this is the analogue of the seminal Kleene’s theorem in the setting of transducers, and second, from a practical perspective, the transducer expressions can form the basis for a declarative language to efficiently evaluate string transformations over finite and infinite words. A key technical contribution here was in identifying the base functions or combinators, the choice of which was driven by the fact that they factorize or parse the domain in an unambiguous manner. Among the two proof directions, the harder one is to start from two way transducers and compute combinator expressions: this part needed a non trivial application of the forest factorization theorem, by considering an unambiguous variant.
Another fundamental question answered in Vrunda’s dissertation relates to checking whether a regular function is computable. This question has long been open; what is known from the literature is how one can check for the computability of rational functions, those given by one way transducers. Vrunda’s work establishes a correspondence between the notions of continuity and computability for regular functions, and provides an algorithm for checking if a regular function is continuous. This required coming up with a “critical pattern” in the runs of transducers corresponding to non continuous functions. Tihs was achieved by considering certain decompositions of the input words and by checking mismatches in their outputs. This forms the core of catching the discontinuity exhibited by the two way transducer. The technique proposed by Vrunda for checking continuity has already been used by others in checking computability of data transducers.
Yet another important contribution of Vrunda’s dissertation relates to the separability problem for string constraints. Vrunda’s main contribution here was in identifying a subclass of string constraints having decidable satisfiability, that subsumes existing classes. She identified this class and provided an encoding of the solutions for this class of string programs to outputs of two way transducers. This helped in reducing the separability problem from string constraints to that of two way transducers.
The ACM India Doctoral Dissertation Award was established in 2011. This award recognizes the best doctoral dissertation from a degree-awarding institution based in India for each academic year, running from August 1 of one year to July 31 of the following year. The ACM India Doctoral Dissertation Award is accompanied by a prize of ₹2,00,000. An Honorable Mention award is accompanied by a prize of ₹1,00,000. The dissertation(s) will be published in the ACM Digital Library. Financial support for both the award and honorable mention is provided by Tata Consultancy Services (TCS). Please see the ACM India Doctoral Dissertation Award page for additional information on current and past winners.
Please join us in congratulating Suhail Sherif, Suprovat Ghoshal and Vrunda Dave.
Regards,
Hemant Pande, Executive Director, ACM India Council
(On behalf of the ACM India Awards Steering Committee)
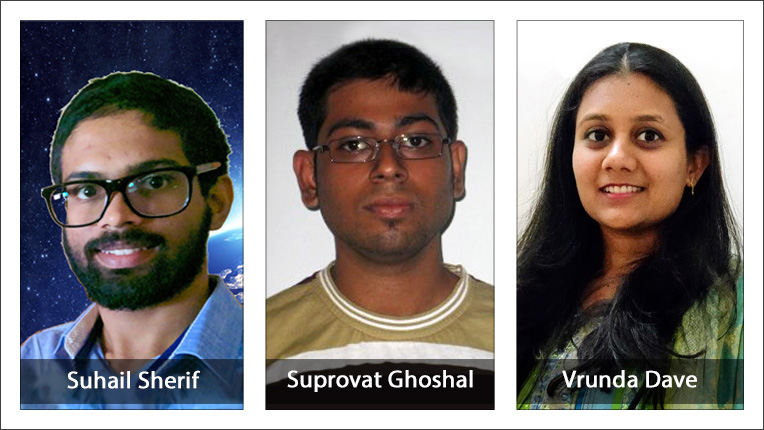