Siddharth Sandipkumar Bhandari Chosen as Recipient of ACM India 2022 Doctoral Dissertation Award
January 31, 2023
Siddharth Sandipkumar Bhandari is the recipient of the ACM India 2022 Doctoral Dissertation Award for his dissertation titled “Exact Sampling and List Decoding” that develops new techniques and tools in sampling graph colourings and contributes to improved analyses for understanding list-decodability of codes. Siddharth’s doctoral dissertation work was done at Tata Institute of Fundamental Research, Mumbai under the supervision of Prahladh Harsha.
Siddharth’s dissertation makes fundamental contributions to two different areas of theoretical computer science: (a) sampling colourings of graphs and (b) list-decoding error-correcting codes. Sampling a random k-colouring of a given graph is a classic problem in theoretical computer science and statistical physics. The problem of perfect sampling is to construct a polynomial time randomised algorithm that generates a perfect sample from the uniform distribution of k-colourings. Siddharth’s work improves on a two-decade old algorithm for solving this problem using novel techniques that are likely to have wider applicability. In the area of list-decoding, Siddharth’s dissertation focuses on three problems. The first problem is the zero-error capacity of the q/(q 1) channel. Prior work on perfect hashing shows that as the list-size is reduced, the channel capacity decreases from an inverse-polynomial form to an inverse-exponential form, and it has been an open problem to understand where this transition occurs. By showing that the channel follows a coupon-collector like behaviour, Siddharth’s work demonstrates that there is an almost sharp transition near O(q log q). The other two results in this part are related to list-decodability of algebraic codes and lead to a better understanding of the list-decoding of these codes all the way up to capacity.
Pritish Mohapatra shares the Honorable Mention for his dissertation titled “Optimization for and by Machine Learning” that makes significant contributions towards the design of efficient optimization methods for machine learning, including key results for optimizing ranking metrics used in information retrieval systems. Pritish’s doctoral dissertation work was done at International Institute of Information Technology, Hyderabad under the supervision of C. V. Jawahar.
Pritish’s dissertation addresses the problem of making optimization of complicated loss functions efficient and practical. Specifically, he designs a practically efficient optimization algorithm for a category of non-decomposable ranking metrics that greatly improve the feasibility of using such sophisticated metrics for learning in information retrieval tasks. Pritish also provides a concrete theoretical analysis that proves the superiority of the proposed algorithm for optimization, providing a fine balance between the theoretical soundness and practical utility of the algorithms. Pritish’s work also makes an interesting contribution in the use of the classical optimization technique of partial-linearization for efficient learning of large-scale classification models. This holds special significance for learning of models with structured output spaces. Finally, Pritish’s dissertation contributes to the problem of using learning for optimization. He proposes a novel framework that uses a learnable model for doing rounding for combinatorial optimization algorithms. This is based on a key insight that randomized rounding procedures can be visualized as sampling from latent variable models.
Sruthi Sekar shares the Honorable Mention for her dissertation titled “Near-Optimal Non-Malleable Codes and Leakage Resilient Secret Sharing Schemes” that makes fundamental contributions in our understanding of cryptographic primitives such as non-malleable codes, secret sharing schemes and randomness extractors. Sruthi’s doctoral dissertation work was done at Indian Institute of Science, Bangalore under the supervision of Bhavana Kanukurthi.
The goal of non-malleable codes (NMCs) is to enable encoding of messages so that an adversary cannot tamper it into the encoding of a related message. An open problem in this area is to build 1/2-rate NMCs in 2-split state model where a codeword consists of two independently tamperable states. Sruthi’s dissertation makes fundamental contributions to this area. Specifically, she builds on her earlier work to introduce new two-state non-malleable codes for random messages with rate 1/2. This work also introduces a new primitive called ``Non-malleable randomness encoders”. Her dissertation also shows how to build a near-optimal rate 1/3, 2-state NMC, thereby taking us a step closer to solving the main open problem in this area. In addition, Sruthi’s dissertation introduces a new pseudorandomness primitive called ``Adaptive Extractors'' and shows their applicability to building constant-rate leakage resilient secret sharing schemes.
Deepika Yadav shares the Honorable Mention for her dissertation titled “Supporting Ongoing Training of Community Health Workers through Mobile-based Solutions in Rural India” that combines the knowledge of Computer System research and HCI to produce systems that are deployed in fields. Her work resulted in deployment of a mobile based training platform for ASHA workers that has been used to train hundreds of ASHA workers. Deepika’s doctoral dissertation work was done at Indraprastha Institute of Information Technology Delhi under the supervision of Pushpendra Singh.
Deepika’s dissertation develops a low-cost mobile-based training platform called “Sangosthi” that allows a geographically distributed group of community health workers (CHWs) to connect over a conference call and receive training in a structured manner. The developed system uses a hybrid architecture to use Interactive Voice Response for facilitating online audio training sessions, enabling CHWs to access training from anywhere through their feature phones. Her work contributes to (i) testing the feasibility and efficacy of a low-cost technology intervention through a controlled field experiment (ii) unpacking the training needs of CHWs in the field and mapping it back to the existing reference material through a large-scale deployment on 500 CHWs, (iii) investigating the potential for peer-to-peer learning models to address the challenge of experts’ availability through a controlled field experiment, and (iv) exploring the potential for automated techniques in this domain by proposing a semi-automated natural language processing approach for curating generated learning content and exposing CHWs and women to Chatbot-based education for the first time. By using a range of mixed methods and field experiments, Deepika’s dissertation expands the focus of HCI4D and mHealth research on CHW competence development in low-resource settings.
The ACM India Doctoral Dissertation Award was established in 2011. This award recognizes the best doctoral dissertation from a degree-awarding institution based in India for each academic year, running from August 1 of one year to July 31 of the following year. The ACM India Doctoral Dissertation Award is accompanied by a prize of ₹2,00,000. An Honorable Mention award is accompanied by a prize of ₹1,00,000 which is shared amongst the recipients. The dissertation(s) will be published in the ACM Digital Library. Financial support for both the award and honorable mention is provided by Tata Consultancy Services (TCS). Please see the ACM India Doctoral Dissertation Award page for additional information on current and past winners.
Please join us in congratulating Siddharth Sandipkumar Bhandari, Pritish Mohapatra, Sruthi Sekar, and Deepika Yadav.
Regards,
Ranjita Bhagwan
(on behalf of ACM India Awards Steering Committee)
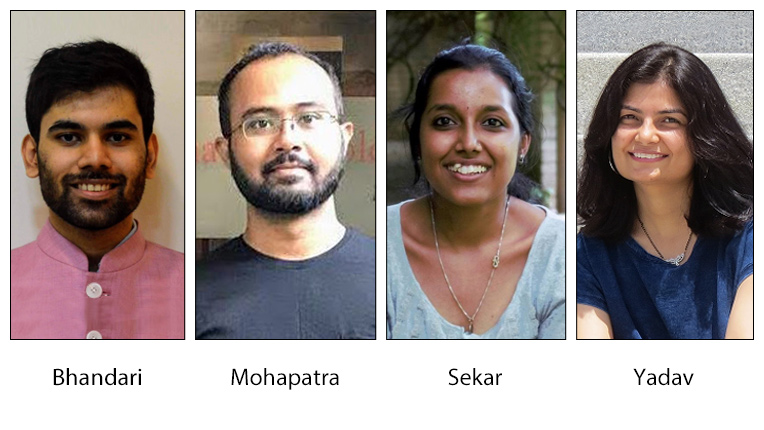