Case Study: Automated Active Response Weaponry
Q Industries is an international defense contractor that specializes in autonomous vehicles. Q’s early work focused on passive systems, such as bomb-defusing robots and crowd-monitoring drones. As an early pioneer in this area, Q established itself as a vendor of choice for both military and law enforcement applications. Q’s products have been deployed in a variety of settings, including conflict zones and non-violent protests.
In recent years, Q has suffered a number of losses, as protestors and other individuals have physically attacked the vehicles with rocks, guns, and other weapons. To reduce this problem, Q has begun to experiment with automated active responses. Q’s first approach was to employ facial recognition algorithms to record those present and to detect individuals who may pose a threat. This approach was shortly followed by automated non-lethal responses, such as tear gas, pepper spray, or acoustic weapons, to incapacitate threatening individuals.
Q has recently been approached—in secret meetings—by multiple governments to expand this response to include lethal responses of varying scales. These capabilities range from targeted shooting of known individuals to releasing small-scale explosives. When Q leadership agreed to pursue these capabilities, several of Q’s original engineers resigned in protest. Some of these engineers had previously expressed concern that the non-lethal responses had inadequate protections against tampering, such as replacing tear gas with a lethal poison. Knowing that these individuals were planning to speak out publicly, Q sued them for violating the terms of their confidentiality employment agreement.
Analysis
Principle 1.2 states that systems designed with the intention of causing harm must be ethically justified and must minimize unintended harm. Q’s evolving work shows a gradual process toward violating this principle. Q’s first automated response employed machine learning in a way that could be used to suppress free speech and association, which are fundamental human rights (Principle 1.1). By failing to account for this risk, Q failed to adhere to Principle 2.5’s mandate of extraordinary care regarding risks in machine learning systems. Furthermore, these tools could be used by governments that do not respect the values expressed in Principle 1.4, allowing discrimination and other abuses. This technology also failed to respect the privacy of other individuals who may be innocent bystanders (Principle 1.6). Additionally, the harm induced by the non-lethal weapons was not minimized, as these weapons would subject innocent individuals to pain or death without due process. Thus, the harm made possible here is not ethically justified and violates Principle 1.2.
The engineers who resigned and decided to speak out would be justified in doing so, based on Principles 1.2, 1.7, and 2.7. The harm that Q’s systems made possible violated several aspects of the ethical obligations of the Code. Thus, the engineers were right to foster public awareness of these risks, and breaking their confidentiality agreements would be ethically justified. At the same time, Q’s lawsuit is justified and the engineers must accept the consequences for breaking these agreements, per Principle 2.3.
Overall, Q’s leadership failed to respect the Code’s overarching emphasis on the public good. The Preamble introduces this emphasis by stating that public good is the paramount consideration. Principle 3.1 reiterates this point, declaring that people should always be the central concern. Q’s leadership failed to adhere to this key tenet that underlies the other principles of the Code.
These case studies are designed for educational purposes to illustrate how to apply the Code to analyze complex situations. All names, buisnesses, places, events, and incidents are fictious and are not intended to refer to actual entities.
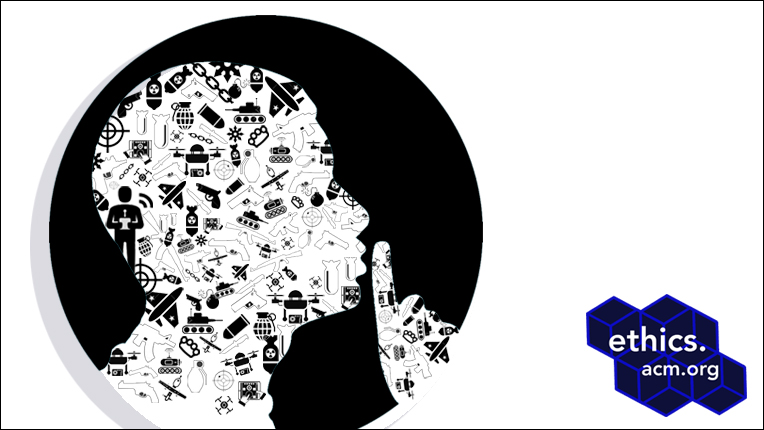
Using the Code: Case Studies
Demonstrating how the principles of the Code can be applied to specific ethical challenges:
- Malware Disruption: Security vendors and government organizations collaborate to disrupt the operation of an ISP that hosts malware.
- Medical Implant Risk Analysis: A medical implant device maker creates a smart phone application to monitor and control the device.
- Abusive Workplace Behavior: A manager fails to address abusive behavior by a technical team leader.
- Autonomous Active Response Weapons: A defense contractor that specializes in autonomous vehicles begins to integrate automated weaponry.
- Dark UX Patterns: A web developer realizes that their client’s requests are intended to trick users into making accidental and expensive purchases.
- Malicious Inputs to Content Filters: An Internet content filtering service deploys machine learning techniques to automate the classification of blocked content.
- Accessibility in Software Development: A web-based collaboration tool deploys a new inline feature that has significant accessibility issues.